As an enterprise data strategy leader, you know the industry is full of buzzwords, and today nothing is receiving attention quite like data mesh — a new paradigm for data management.
Per a Google Trends report, queries for “data mesh” in 2021 surged, even surpassing searches for “data lakehouse.” Ellie Technologies even states the Slack channel, “Data Mesh Learning,” is growing faster than most data-focused sites.
The concept of data mesh originated in 2018 and is credited to Zhamak Dehghani, Director of Emerging Technologies at ThoughtWorks, who describes data mesh as, “an analytical data architecture and operating model where data is treated as a product and owned by teams that most intimately know and consume the data.”
Early adopters of data mesh include Netflix and other tech-savvy enterprises, and today there are a growing number of companies who have implemented or are planning to implement data mesh.
Data mesh may be the talk of the town, but is it worth the hype? More importantly, is it the right move for your program and enterprise?
The problems addressed, and created, by data mesh
Amid the push for digital transformations, organizations have quickly scaled to unlock the potential of enterprise data by providing key decision-makers with quick time-to-insights.
However, Zhamak suggests in her 2022 book titled Data Mesh that most enterprises are still struggling to empower and enable their employees to leverage data to make the most informed and timely decisions possible. She argues this is the result of centralized data platform architectures that fail to deliver insights quickly and with the flexibility most organizations need.
Zhamak, and many others, see data mesh as a solution to this challenge by shifting responsibility to those enterprise members who are closest to the data, thus enabling its scalability.
In a VentureBeat article, dbInsight Principal Tony Baer writes, “[Data mesh is] a topic that addresses a point of pain: We dump all sorts of data into data lakes or other silos, then we lose track of them or don’t adequately utilize and govern them.”
He explains that data mesh addresses several valid concerns about the limitations of top-down management and data ownership, but argues the concepts are not fully fleshed out, particularly when it comes to self-service or governance.
The concept of data mesh suggests that the domains — or business units — with the highest subject matter expertise should own the data and manage it from start to finish, theoretically improving management and accountability. Yet, Tony outlines some potential pitfalls with this model.
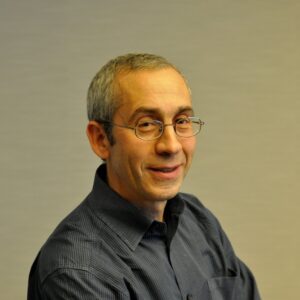
“The downside is that not properly managed, data meshes could amplify or proliferate data silos, leading to waste, duplication, and inconsistent management and governance.”
Tony Baer, dbInsight Principal
Zhamak acknowledges some of these challenges in an article she wrote for Martin Fowler. She says these issues exist with preexisting data architectures, along with the high friction and cost of discovering, understanding, trusting, and using quality data.
“If not addressed, this problem only exacerbates with data mesh, as the number of places and teams who provide data — domains — increases,” she said, adding this is the consequence of decentralization.
However, there are some cases when data mesh can be effective, particularly in organizations that already treat data as a product.
While data mesh may not be scalable across the entire enterprise, there are instances where it could prove effective.
Tony suggests data mesh should be implemented at a modest scale, specifically across teams that share overlapping subject matter expertise such as customer expertise and product development.
Hype vs. practical implementation
It’s no secret that data strategy is an ever-evolving space, and when new concepts emerge it can be difficult to distinguish between what’s hype and what’s helpful.
When you’re faced with hurdles like this, it’s helpful to benchmark with a group of trusted peers. Members of the Enterprise Data Strategy Board have weekly confidential discussions to share their experiences on topics like data mesh — the problems it can solve and create.
Without any vendors or consultants on the line, senior data leaders at multibillion-dollar brands share how they’re operationalizing a data mesh design, what reporting structure they’re leveraging to make it successful, and more in our community.